Importance of Clinical Data with Biospecimens
Published On 10/16/2024 6:01 PM
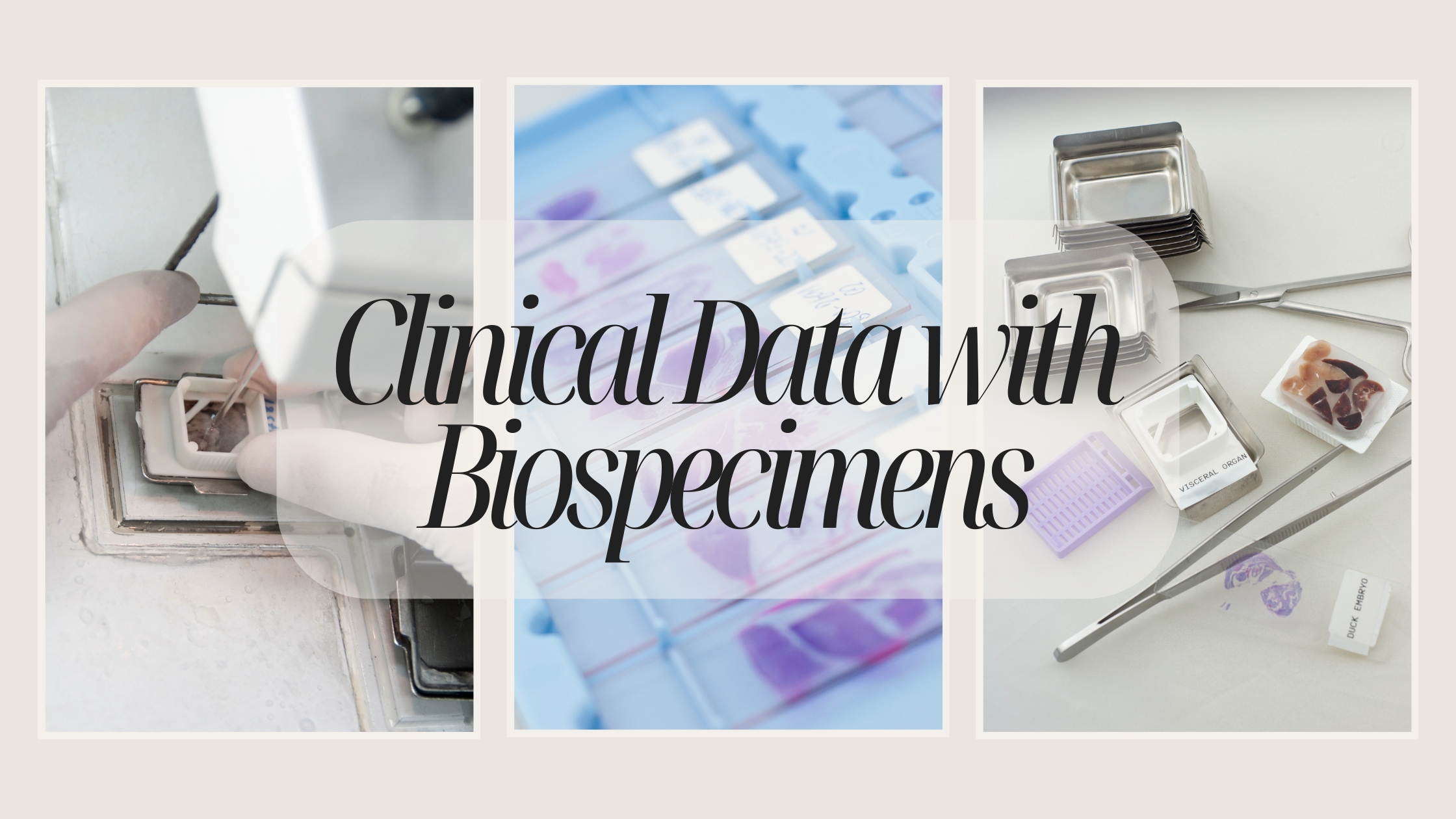
Discover why clinical data is crucial for biospecimen research. Learn about the essential components of high-quality clinical data, practical strategies for effective use, and how integrating clinical data can enhance biomedical research outcomes.
Data is collected consistently using standardized terminologies and coding systems, ensuring that all relevant variables are captured and easily integrated into the research workflow.
Imaging data, such as MRI or CT scans, is provided alongside lab results, giving a complete view of disease progression and treatment response.
Clinical outcomes are not systematically recorded, making it difficult to correlate biospecimen findings with patient prognosis. The lack of standardized data collection leads to inconsistencies that hinder meaningful analysis.
Imaging data is absent, and laboratory results are not documented with sufficient detail, limiting the ability to draw conclusions from biospecimen analysis.
Addressing the challenges of collecting and integrating clinical data—such as ensuring privacy, data quality, and interoperability—is essential for maximizing the potential of biospecimens in advancing biomedical research. By adopting practical strategies and leveraging technological advancements, researchers can unlock the full potential of clinical data to drive innovation in healthcare.
Importance of Clinical Data with Biospecimens
In the realm of biomedical research, biospecimens such as blood, tissue, and other biological materials are pivotal in advancing our understanding of diseases, drug responses, and personalized medicine. However, the value of biospecimens is significantly enhanced when combined with detailed clinical data. Clinical data provides essential context, allowing researchers to draw more meaningful conclusions, identify biomarkers, and develop targeted therapies. This article explores the importance of clinical data in biospecimen research, what constitutes high-quality clinical data, and practical insights into evaluating and integrating this data effectively.The Role of Clinical Data in Biospecimen Research
Clinical data refers to any health-related information collected from patients, such as medical history, demographics, treatment outcomes, and laboratory test results. When paired with biospecimens, this data provides a comprehensive view of the patient’s health status, which is crucial for understanding disease mechanisms and developing effective treatments. Here are several reasons why clinical data is critical in biospecimen research:Enhancing Research Outcomes: Biospecimens without clinical data are limited in their utility. Clinical data provides the context needed to interpret the biological information contained within biospecimens, enabling researchers to understand the relationship between molecular changes and clinical symptoms.
Advancing Personalized Medicine: Clinical data allows for the identification of patient-specific factors, such as genetic mutations or comorbidities, which influence how individuals respond to treatment. This data is essential for developing personalized therapies that are tailored to the unique characteristics of each patient.
Biomarker Discovery: Identifying biomarkers for early disease detection or treatment response requires a detailed understanding of patient characteristics and disease progression. Clinical data is fundamental to correlating changes in biospecimens with clinical outcomes, facilitating the discovery of meaningful biomarkers.
Advancing Personalized Medicine: Clinical data allows for the identification of patient-specific factors, such as genetic mutations or comorbidities, which influence how individuals respond to treatment. This data is essential for developing personalized therapies that are tailored to the unique characteristics of each patient.
Biomarker Discovery: Identifying biomarkers for early disease detection or treatment response requires a detailed understanding of patient characteristics and disease progression. Clinical data is fundamental to correlating changes in biospecimens with clinical outcomes, facilitating the discovery of meaningful biomarkers.
Components of High-Quality Clinical Data
To maximize the research potential of biospecimens, the accompanying clinical data must be of high quality. High-quality clinical data includes the following components:Patient Demographics: Information such as age, gender, ethnicity, and lifestyle factors (e.g., smoking status, diet) provides a basis for understanding how these variables influence disease risk and treatment response.
Medical History: Detailed medical history, including past illnesses, family history, and current health conditions, helps contextualize the biological data derived from biospecimens.
Disease Characteristics: Information on the type of disease, its stage, duration, and specific symptoms is essential for understanding disease mechanisms and tailoring research approaches.
Treatment and Intervention Data: Data on current and past treatments, including drugs, dosage, and response to treatment, allows researchers to study the effectiveness of different therapies and their impact on patient health.
Clinical Outcomes: Measurable outcomes, such as progression-free survival or response rates, provide insights into the effectiveness of treatments and help correlate molecular findings with patient prognosis.
Laboratory Results and Imaging: Diagnostic test results, imaging findings, and laboratory reports are key components that provide additional layers of information, enriching the interpretation of biospecimen analysis.
Medical History: Detailed medical history, including past illnesses, family history, and current health conditions, helps contextualize the biological data derived from biospecimens.
Disease Characteristics: Information on the type of disease, its stage, duration, and specific symptoms is essential for understanding disease mechanisms and tailoring research approaches.
Treatment and Intervention Data: Data on current and past treatments, including drugs, dosage, and response to treatment, allows researchers to study the effectiveness of different therapies and their impact on patient health.
Clinical Outcomes: Measurable outcomes, such as progression-free survival or response rates, provide insights into the effectiveness of treatments and help correlate molecular findings with patient prognosis.
Laboratory Results and Imaging: Diagnostic test results, imaging findings, and laboratory reports are key components that provide additional layers of information, enriching the interpretation of biospecimen analysis.
Evaluating Clinical Data for Research
The value of biospecimen research is highly dependent on the quality of the clinical data that accompanies it. To ensure that clinical data can be effectively used in research, several factors must be considered:Data Quality: High-quality data is complete, accurate, and reliable. Incomplete or incorrect data can lead to biased research findings. Ensuring data quality requires careful collection, validation, and standardization processes.
Consistency and Standardization: Clinical data should be collected using standardized terminologies, such as ICD codes for diagnoses, to facilitate integration and comparison across different studies. Consistency in data collection methods helps ensure that the data can be effectively utilized for large-scale research.
Relevance to Research Objectives: The clinical data collected should be directly relevant to the research objectives. For example, a study on cancer biomarkers should include data on tumor characteristics, treatment regimens, and patient outcomes to provide meaningful insights.
Consistency and Standardization: Clinical data should be collected using standardized terminologies, such as ICD codes for diagnoses, to facilitate integration and comparison across different studies. Consistency in data collection methods helps ensure that the data can be effectively utilized for large-scale research.
Relevance to Research Objectives: The clinical data collected should be directly relevant to the research objectives. For example, a study on cancer biomarkers should include data on tumor characteristics, treatment regimens, and patient outcomes to provide meaningful insights.
Challenges in Collecting and Integrating Clinical Data
Collecting and integrating clinical data with biospecimens presents several challenges, including:Data Privacy and Compliance: Protecting patient privacy is paramount, and researchers must adhere to regulations such as GDPR and HIPAA. Obtaining informed consent from patients and ensuring data is anonymized are critical steps in maintaining compliance.
Data Completeness and Variability: Clinical data is often incomplete, especially when collected from different healthcare providers. Variability in the type and quality of data available can pose challenges in integrating and analyzing the information effectively.
Interoperability: Integrating clinical data from different healthcare systems can be challenging due to differences in data formats, collection methods, and healthcare IT infrastructure. Ensuring interoperability requires the use of standardized data formats and robust data integration solutions.
Data Completeness and Variability: Clinical data is often incomplete, especially when collected from different healthcare providers. Variability in the type and quality of data available can pose challenges in integrating and analyzing the information effectively.
Interoperability: Integrating clinical data from different healthcare systems can be challenging due to differences in data formats, collection methods, and healthcare IT infrastructure. Ensuring interoperability requires the use of standardized data formats and robust data integration solutions.
Practical Strategies for Effective Use of Clinical Data
To maximize the value of clinical data in biospecimen research, researchers can adopt several practical strategies:Stakeholder Collaboration: Collaborating with healthcare providers, data managers, and IT teams is essential to ensure seamless data collection and integration. Engaging stakeholders early in the project can help address data-related challenges and improve the quality of collected data.
Data Collection Tools: Leveraging electronic health records (EHRs), patient-reported outcomes (PROs), and mobile health (mHealth) devices can streamline the collection of relevant clinical data. These tools help automate data collection, reduce manual errors, and improve data completeness.
Quality Control Measures: Implementing quality control protocols, such as data validation checks and regular audits, helps ensure the accuracy and consistency of clinical data throughout the research project. Quality assurance measures are crucial for maintaining the integrity of research findings.
Data Collection Tools: Leveraging electronic health records (EHRs), patient-reported outcomes (PROs), and mobile health (mHealth) devices can streamline the collection of relevant clinical data. These tools help automate data collection, reduce manual errors, and improve data completeness.
Quality Control Measures: Implementing quality control protocols, such as data validation checks and regular audits, helps ensure the accuracy and consistency of clinical data throughout the research project. Quality assurance measures are crucial for maintaining the integrity of research findings.
Clinical Data: Good vs. Bad
Good Clinical Data:
A cancer research project includes biospecimens from patients with detailed clinical data, such as precise tumor stage, specific genetic mutations, comprehensive treatment history, and measured clinical outcomes like response rates and progression-free survival.Data is collected consistently using standardized terminologies and coding systems, ensuring that all relevant variables are captured and easily integrated into the research workflow.
Imaging data, such as MRI or CT scans, is provided alongside lab results, giving a complete view of disease progression and treatment response.
Bad Clinical Data:
Biospecimens are collected from patients, but the accompanying clinical data is incomplete or inconsistent. For example, patient treatment history is missing or lacks specific dosage information, and only general disease descriptions are available without details on disease stage.Clinical outcomes are not systematically recorded, making it difficult to correlate biospecimen findings with patient prognosis. The lack of standardized data collection leads to inconsistencies that hinder meaningful analysis.
Imaging data is absent, and laboratory results are not documented with sufficient detail, limiting the ability to draw conclusions from biospecimen analysis.
Conclusion
Clinical data is indispensable in biospecimen research, providing the context needed to understand biological processes, identify biomarkers, and develop personalized treatments. High-quality clinical data includes detailed patient demographics, medical history, treatment information, and clinical outcomes. By effectively evaluating and integrating clinical data, researchers can enhance the value of biospecimens, ultimately leading to more meaningful research outcomes and improved patient care.Addressing the challenges of collecting and integrating clinical data—such as ensuring privacy, data quality, and interoperability—is essential for maximizing the potential of biospecimens in advancing biomedical research. By adopting practical strategies and leveraging technological advancements, researchers can unlock the full potential of clinical data to drive innovation in healthcare.
This entry was posted in
Biospecimen